4. Data Acquisition, Transmission, and Resource Management
In the radar network to be considered in KoRaTo, due to the large bandwidth of radar signals and high-resolution A/D conversion, the amount of data generated in the mobile sensors is enormous. The question is, which data have to be collected, how redundancies can be removed, and which parts of the collected data have to be communicated. From the raw data, the information of interest has to be extracted by aggregation of the partial signals of the individual sensors and signal processing. With respect to the final result, the partial signals have a very low information content. Furthermore, since the relevant contributions of the partial signals are only known after joint processing, classical compression techniques (source coding) are not immediately applicable. However, each aggregation of the radar data collected by the distributed drones requires the transmission of enormous amounts of data over a time-varying radio channel. Hence, the additional question arises at which point in the distributed sensor system (centralized/decentralized), at which point in time (online/offline), and in which way (high-frequency electromagnetic/optical) the different parts are to be aggregated and the imaging should take place. Thereby, it has to be considered that the drones have limited computational and storage capabilities and limited energy resources compared to a central station.
Therefore, in KoRaTo application-specific methods are to be developed in order to represent the data compactly, to exchange them at low cost, and to operate the network efficiently.
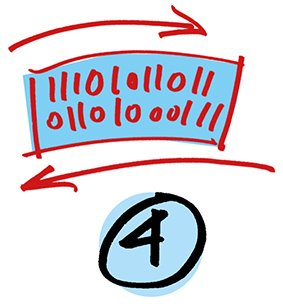
Sub-Projects
Distributed Compressed Sensing for Cooperative Aperture Systems
Lead: Prof. Dr.-Ing. Robert Fischer, Institute of Communications Engineering, UUlm
In the cooperative aperture system, the sensing in the individual UAVs (drones) and the data communication must be very carefully matched. In a first approach, we consider how compressed sensing techniques can be used in the distributed setting. As each drone performs only partial measurements, a distributed compressed sensing problem is present. Depending on the trajectory of the UAVs, the acquired signal may be highly correlated. For the design of the reconstruction algorithms, a statistical model of the radar data must be extracted from measurement data generated by the partners. Assuming powerful, especially iterative algorithms, the reconstruction quality in case of central processing is to be determined. The influence of the resolution of the A/D conversion and the signal quality on the channel shall be quantified. In a second step, a successive aggregation of the measurement data shall be considered. E.g., in a tree structure, drones collect data from their neighbors and combine them with their measurements. Thereby, not only the reconstruction quality of the raw data by compressed sensing is of interest, but the quality of the final radar evaluation.
Optimization of Resource Allocation and Communication
Lead: Prof. Dr.-Ing. Robert Schober, Institute for Digital Communication, FAU
The resources available for data acquisition (sensing), communication, and operation of UAVs are severely limited due to the bandwidth limitation of the communication channel and the autonomous power supply of the UAVs. Furthermore, enormous amounts of data are generated by the individual mobile radar sensors, which, depending on the operating mode, have to be forwarded to other UAVs and/or a ground station. Therefore, the optimal allocation of the available resources, such as energy, bandwidth, antennas, and UAV trajectory, is of great importance for the quality and quantity of the radar image.
To optimize resource allocation and communication in drone-based radar systems, we will will work towards the following objectives:
-
Optimal resource planning for multi-static drone-based radar networks: Suitable concepts for the joint optimization of sensing, data transmission and trajectory are to be investigated. For this purpose, appropriate models are to be developed and optimization problems with suitable objective functions and constraints are to be formulated and solved.
-
Channel modeling and conception of free-space optics (FSO) systems for mobile drone networks: Realistic FSO channel models for the connections between mobile drones and from mobile drones to a ground station are to be developed. In addition, new transmission concepts for FSO systems for mobile drone networks are to be studied.
-
Energy harvesting concepts for drone-based radar networks: In order to extend the service life of drone-based mobile radar networks, energy harvesting concepts are to be examined and optimized together with sensing, data transmission, and trajectories.