SleepLoop ist ein Flagship der Hochschulmedizin Zürich bestehend aus Forschern aus Zürich (ETH Zürich, Universität Zürich, Kinderspital Zürich, Universitätsspital Zürich) und Ulm (Institut für Biomedizinische Technik). Das Ziel dieser Teams ist es mit technischen und klinischen Innovationen den Schlaf besser zu erforschen. Dafür werden auch wearables, Big Data, und auditive Stimulation eingesetzt. Mit diesem Ansatz sollen bessere Methoden für die Behandlung und Prävention von Hirn- und Systemerkrankungen entwickelt und validiert werden.
Wieso Schlaf?
Schlaf gehört zu den Grundbedürfnissen des Menschen. Wir müssen schlafen, um zu überleben. Ausserdem dient der Schlaf der Hirnentwicklung, der „Reinigung“ des Gehirns, dem Lernen und letztendlich der Gesundheit. Dem gegenüber steht die Tatsache, dass Schlafstörungen zunehmen und dass ungenügender und unregelmässiger Schlaf in der Gesellschaft sehr weit verbreitet sind.
Wie verbessert man den Tiefschlaf?
Tiefschlaf kann mittels Tönen, die auf Hirnwellen synchroniert abgespielt werden, verstärkt werden. Diese Methode kann auch ausserhalb des Schlaflabors angewendet werden und funktioniert sowohl bei Jung und Alt. Viele Aspekte und Mechanismen zu dieser Methode sind noch nicht erforscht. Deshalb entwickeln wir diese Technologie weiter und erforschen mit Langzeitstudien deren Effekt auf Körper und Gesundheit.
Wie funktioniert die SleepLoop Technologie?
Unsere Projekte
SleepBand
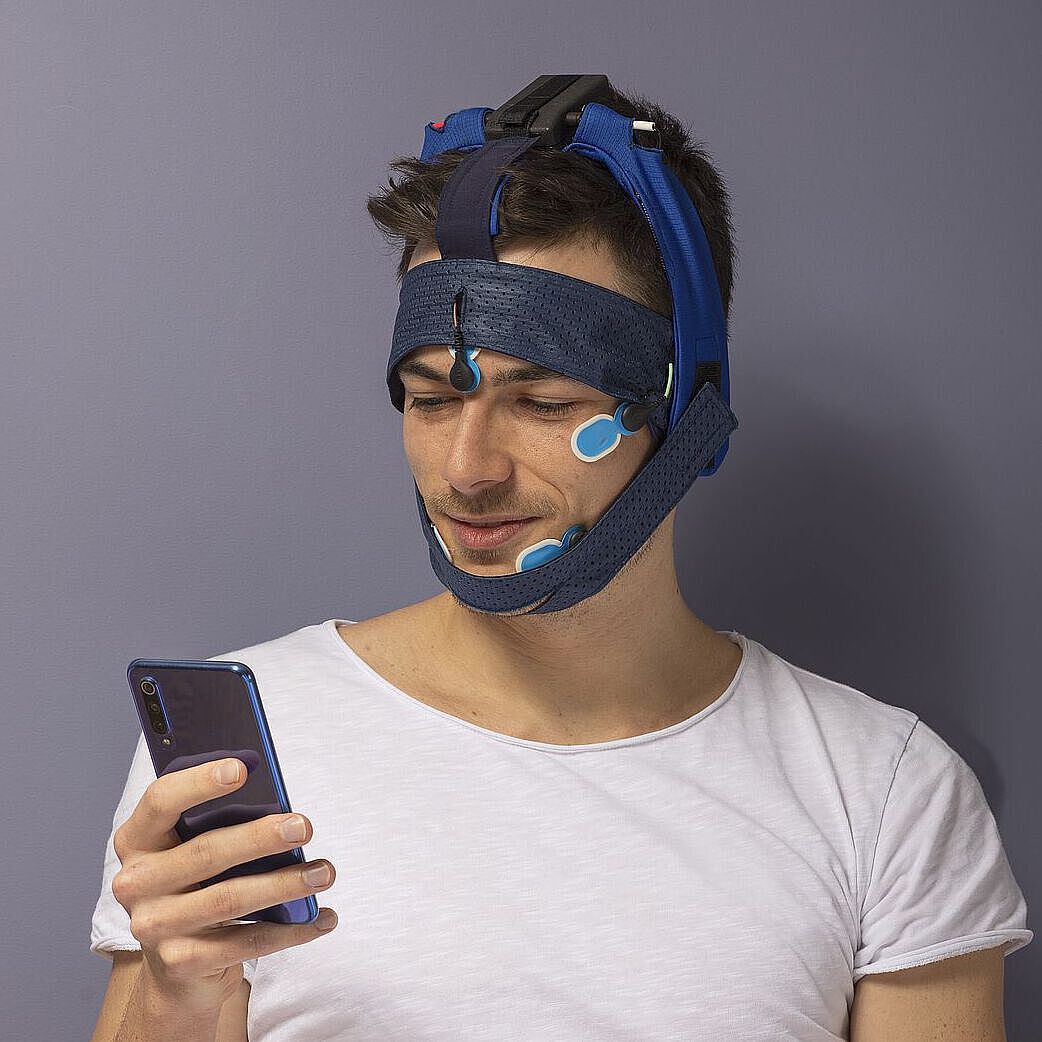
Our mission is to develop wearables with personalized sleep enhancement solutions to enable large-scale research studies and future clinical treatment strategies. With our technology we enable safe brain activity recording and stimulation with superb temporal precision during selected sleep stages. Our autonomous algorithms use the underlying brain activity to control and optimize stimulation to maximally enhance brain activity during deep sleep.
Selected Key features:
- Low-Noise, up to 8-Channel, 24-Bit, Analog-to-Digital Converter for EEG and biopotential measurements
- Ultra-low-power ARM Cortex-M4 for real-time signal processing.
- Rechargeable battery with up to 24 h of recording
- Internet-of-things ready: Bluetooth and Wi-Fi connectivity
- Raw data recording to microSD Card
Publications:
Ferster ML, et al. Configurable Mobile System for Autonomous High-Quality Sleep Monitoring and Closed-Loop Acoustic Stimulation. IEEE Sensors Letters. 2019; 3(5):1--4.
WESA
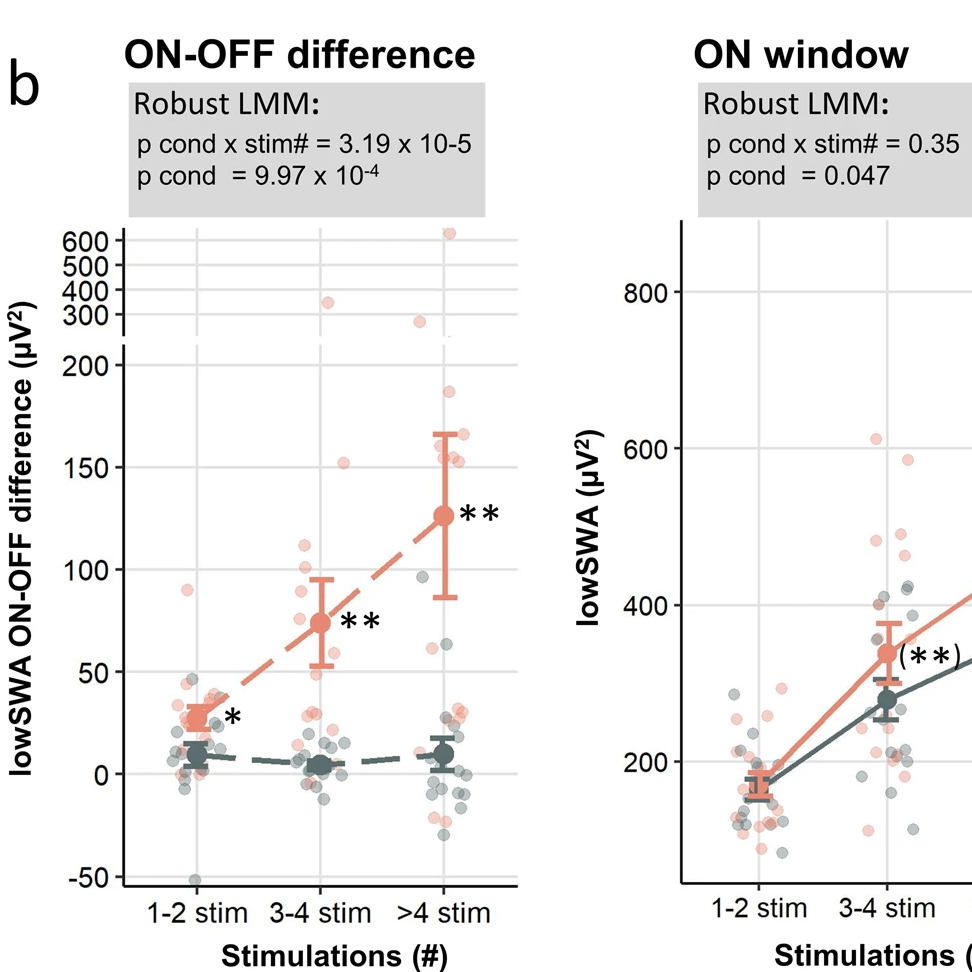
Sleep’s restorative function is closely linked to slow waves, which are brain activity patterns that occur during deep sleep and are diminished with age. Those slow waves can be increased through auditory stimulation, a method that administers precisely-timed sounds during sleep. Here, we established whether the application of auditory stimulation can be performed in older adults over several nights remotely in their own homes. In a trial, we used a mobile device to deliver auditory stimulation during sleep and measured its effects on slow waves, mood, and vigilance. Although we showed robust increases in slow waves, we found large effect differences between participants and also between different nights within the same participants. We looked for predictors of these effect differences. Our study showed that in-home auditory stimulation is feasible, and may help to guide future auditory stimulation strategies.
Publication:
Lustenberger C, et al. Auditory deep sleep stimulation in older adults at home: a randomized crossover trial. Communications Medicine. 2022; 2(1):30. [Doi]
Automated Sleep Classification
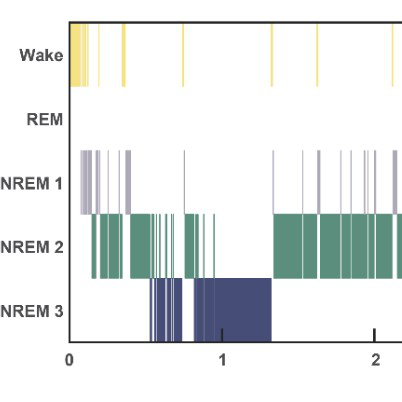
The classification of segments of sleep into one of the five sleep stages – wake, rapid eye movement (REM) sleep , and three non-REM sleep stages – is a standard task in sleep research that is often still performed manually. Distribution of sleep stages enables clinicians and researchers to make valuable observations and assessments regarding sleep architecture, quality and possible disorders. However, the manual sleep classification is labor intensive, costly and can only be applied on selected nights of interest.
To support large studies with automated sleep classification, we have developed a machine learning sleep classification system that is tailored to the SleepLoop recording device. Our method fuses the predictions from multiple deep learning models to produce high-quality labels. Additionally, we investigate alternative classification paradigms such as combining manual and automatic classification in a human-in-the-loop approach.
Our experiments aim to gain a better understanding of the automatic classification quality beyond simple performance metrics, as these are often unsuitable due to the uncertain ground truth present in sleep classification.
Finanzierung
Unsere Arbeiten werden oder wurden durch die
- Schweizerische Hirnstiftung,
- Hochschulmedizin Zürich,
- ETH foundation,
- Schweizer Nationalfond
und weiteren privaten Donatoren unterstützt.
Partner
Unsere Hochschulpartner sind
- ETH Zurich
- University of Zurich
- University Hospital Zurich
- University Children’s Hospital
- Psychiatric University Clinics of Zurich.