Predictive monitoring can support students during the semester by motivating them if they are not performing well enough in a lecture or exercise. Furthermore, supervisor can create additional exercise sheets or can adapt their lectures and exercises to the needs (i.e. knowledge gaps) of the students. To realise this, three different regression algorithms (i.e. Neuronal Networks, Decision Trees, and Random Forest) are implemented to continuously predict further exercise points and the final grade during a semester. These algorithms are trained and tested based on student points and grades from previous semesters. A total of 17,136 predictions were determined, analysed, and compared. In several exercise sheets, all algorithms achieve between 91% and 96% correct predictions with a variance of 10% (i.e. up to 2.5 points). With 15% variance, 98.5% corrected results are possible. The prediction of grades with a variance of 0.3 (i.e. one grade level) with the Decision Tree and the Random Forest only achieves 32% to 35% correctness.
Predictive Process Monitoring: An Implementation and Comparison of Student Performance Prediction
Ulm University Ulm University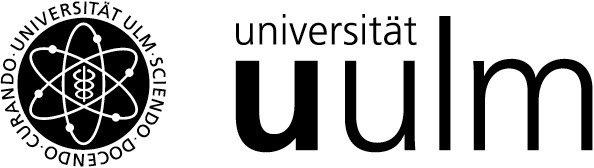
Presentation at the ZEUS; Lisa Arnold, Ulm, Germany, 1 March 2024, 10:30 AM