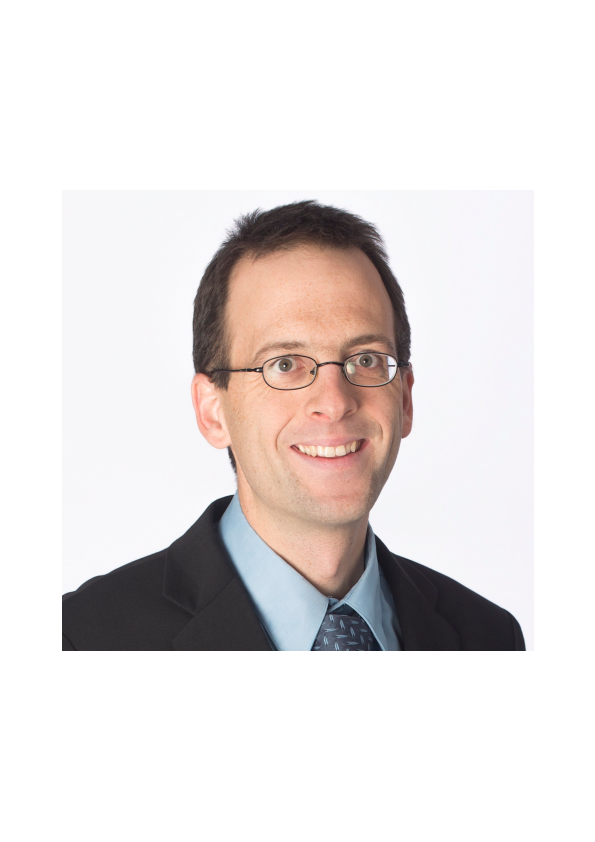
Time & Date: 10.01.2019
5-7 ct
Room 47.0.501 (Teaching block WWP)
Universität West
Albert-Einstein-Allee 47
89081 Ulm
Links:
Cognitive Systems and Human-Computer Interaction
Cognitive Systems M.Sc.
Abstract. An ever-increasing body of experimental literature shows that the brain integrates sensory information in a Bayesian optimal way leading to the so-called Bayesian brain hypothesis. However, most of those evidences come from experiments with static stimuli whereas natural stimuli are intrinsically dynamic. Indeed, the brain is exceptionally good at dynamically estimating hidden features from on-going stimuli such as tracking the position of the prey from on-going visual inputs or identify the voice of a person in noisy crowd. The Bayesian optimal way to perform this on-going feature extraction from dynamical stimuli is known as nonlinear filtering. Here, I propose a generalised hypothesis - the filtering brain hypothesis - which generalises the Bayesian brain hypothesis to dynamic stimuli. In this talk, I will show that this hypothesis can be seen as a guiding computational principle at different levels of descriptions. At the single synapse level, short-term plasticity can be seen as a nonlinear filtering process [1]. At the neural network level, we proposed a new particle filter called the Neural Particle Filter which can be implemented by a neural network [2] and scales well with the dimensionality of the problem unlike traditional particle filters [3].
[1] Pfister, J.-P., Dayan, P., & Lengyel, M. (2010). Synapses with short-term plasticity are optimal estimators of presynaptic membrane potentials. Nature Neuroscience, 13(10), 1271–1275.
[2] Kutschireiter, A., Surace, S. C., Sprekeler, H., & Pfister, J.-P. (2017). Nonlinear Bayesian filtering and learning: a neuronal dynamics for perception. Nature Scientific Reports, 7(1), 8722.
[3] Surace, S. C., Kutschireiter, A., & Pfister, J.-P. (2018). How to avoid the curse of dimensionality: scalability of particle filters with and without importance weights. SIAM Review, in Press, arXiv:1703.07879
Bio. Jean-Pascal Pfister is assistant professor at the Department of Physiology (University of Bern) and at the Institute of Neuroinformatics (University of Zurich / ETH). He is heading the theoretical neuroscience group and aims at discovering fundamental computational principles that govern brain dynamics. During his PhD at EPFL, he developed several models of spike-timing dependent plasticity (including the “triplet model”). During his post-doc in Cambridge (UK) and his sabbatical in Harvard he developed statistical models of synaptic dynamics, neuronal dynamics and neural network dynamics.
Time & Date: 10.01.2019
5-7 ct
Room 47.0.501 (Teaching block WWP)
Universität West
Albert-Einstein-Allee 47
89081 Ulm
Links:
Cognitive Systems and Human-Computer Interaction
Cognitive Systems M.Sc.